In the ancient times of classical Greek myths, there was a nymph. A young, beautiful nymph by the name Echo- a nymph who loved gossip, enough so that Zeus tasked her with keeping his wife Hera busy, whilst he sneaked off to one of his many lovers. However, having found out, Hera punished her- by forcing her to only speak by repeating the last few words of whatever sentence she heard.
Now, this may be a nice story, a mythos to explain how ‘echoes’ originate, but what if it was real? And what if instead of just one person, it was an entire group of people who could only repeat what the others were saying?
Sounds far-fetched? It isn’t.
The concept of an echo chamber is after all just that—a community wherein the same opinions are bounced around, endlessly ‘echoing’ with barely any change. And as they do, any other opinion is shunned, pushed aside, and eventually just rejected without consideration (definition based on the following two sources: Digital Media Literacy: What is an Echo Chamber?, M. Cinelli, G. De F. Morales, A. Galeazzi, W. Quattrociocchi, & M. Starnini). It may sound childish, perhaps, a needless worry. Surely, such things only happen in a conversation between young children, and adults are open to discussion and debate, right?
Alas, that is not so. Echo chambers have formed and have distorted public opinion many times. One case of this happening that the reader may be familiar with is that of the ‘anti-vaxxers’, people who proclaim vaccines to be ineffective and harmful; however, groups like these have existed long before that.
Real-life impact
The incident that led echo chambers to become a notorious phenomenon was in 2016 when upon the presidential elections in the US, the popular social media platform Facebook was reported as having majorly swayed the result in favor of Republican candidate Donald Trump, if unintentionally (Cornell University (2016), Information Cascades and Echo Chambers in the 2016 Presidential Election).
How was such a feat accomplished- and how could it have been unintentional? Facebook uses an algorithm that aims to maximize user engagement with the platform (to put it more simply, to try and get the user to stay on Facebook as much as possible). To do this, the platform would assign ‘tags’ to these users, and only showed them posts containing said tags.
These tags could be anything from your preferred movie, to even your political sympathies. And in doing so, Facebook’s algorithm created a situation where users would only be shown posts that confirmed what they already believed without ever challenging it: thereby creating an echo chamber.
This situation helped Trump more than his opponent. His campaign was founded on an ‘us vs them’ narrative after all, which echo chambers obviously serve only to reinforce. And what’s more, said narrative could not be countered as even his opponents were not fully aware of how much it had spread- again due to being trapped in their own echo chamber!
It bears repeating, that this was in 2016, and it affected the future of one of the world’s largest superpowers. In today’s world, a world that is more ‘online’ than ever before, echo chambers can and do represent one of the primary ways by which misinformation thrives and is spread. Furthermore, they encompass many more situations than just the US elections (for instance, affecting public opinion on the impeachment of the former Brazilian President Dilma Rousseff).
For all the reasons above, we believe echo chambers can form a serious threat leading to polarisation, a threat we need to be aware of and deal with as soon as possible. In the last years, many people have been investigating the formation of echo chambers in different social media platforms during various political and social circumstances around the world. In what follows we will discuss how network science could help understand and detect the formation of an echo chamber.
Network Science
Until now we discussed echo chambers relying on an intuitive approach based on our daily experiences. But as we will argue later on, it can be helpful to also adopt a more formal, mathematical approach to describe them. To do this we rely on network science.
Network science is a general term for the research field that employs networks to understand many phenomena where interactions play a crucial role. The examples are ample, think of the spread of the coronavirus, or the transmission of (mis)information on social media. Two seemingly different phenomena, but both are the result of human interactions.
In what follows, we will first discuss some basic principles and terminology from network science to be able to describe echo chambers, and afterwards, we will lay out some well-established social ‘rules’ that are thought to underlie the formation of such echo chambers.
To start with, what is a network? The essential building blocks of networks are nodes and links; where a node represents an object (such as a person) and a link represents a relationship between nodes (such as two or more people being friends). This framework allows us to define a community: a cluster (or group) of nodes that have many links within the same group, but few to other groups.
An echo chamber is a special type of community, where opinions play an important role. Notably, opinions that are in line with the beliefs of an echo chamber are encouraged, while dissimilar opinions are rejected. Although this may sound simple enough, it is difficult to quantify these concepts or even to compare two echo chambers. In the figure below you can see two visualizations of networks of retweets on two controversial topics. In both networks, this echo-chamber effect on Twitter can be visually observed. Red and blue nodes represent users with opposing beliefs and edges represent who-retweets-whom. Both visualizations and the analysis of the Twitter datasets are due to Kiran Garimella, Gianmarco De Francisci Morales, Aristides Gionis. (2018). Reducing Controversy by Connecting Opposing Views.
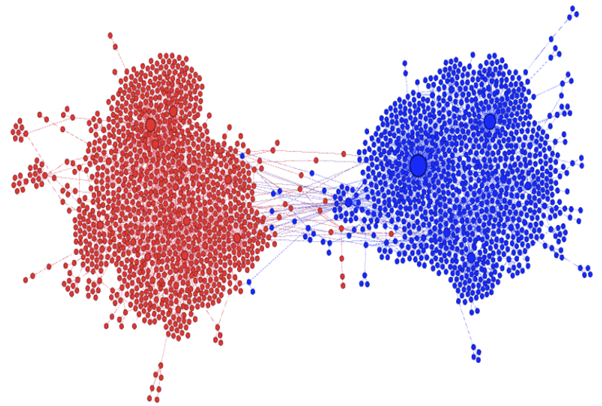
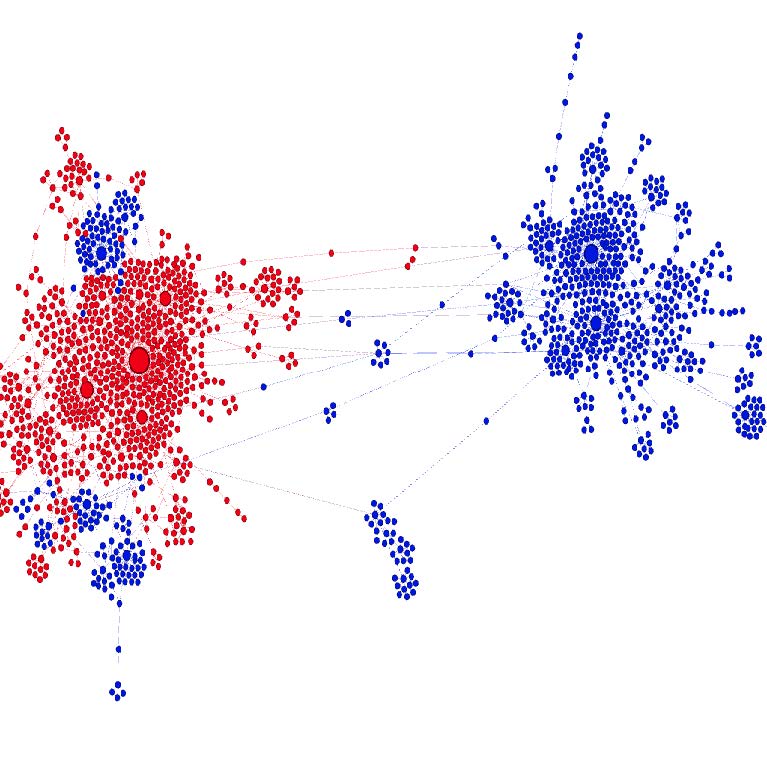
Visualizations of networks of retweets on two controversial topics.
Before discussing concretely how we could construct a mathematical model to study echo chambers we want to make an intermezzo to the psychological aspects behind their formation.
Opinion Reinforcement
An important social aspect-one of the social rules mentioned before- of echo chambers is that the people in such a group share a common opinion, such as left- or right-wing political leanings. Several researchers (such as Ravandi et al.) have suggested that this common opinion is the main reason why these groups can exist in the first place. In other words, people want to be surrounded by people with similar beliefs. And when such a situation occurs, people will only come in contact with opinions that resemble their own. This will most likely then strengthen their own -and their friends’- opinions on this topic, due to a phenomenon known as confirmation bias (see e.g. Wang et al.).
The term confirmation bias comes from psychology and refers to the observation that people are happier to receive information that they already agree with. This effect is exacerbated by recommendation algorithms that many social media platforms employ. These algorithms filter the posts that a user gets to see (their ‘feed’) and will mostly present posts of friends and people with similar opinions. As a result, you will be more likely to engage with these posts and spend more time on the platform.
Researchers that have investigated this effect of opinion reinforcement generally suggest that to combat this effect, it is important to make the kind of posts that people interact with on social media more diverse in opinion (Feldman et al.). This is in direct contrast to the recommendation algorithms at the very foundation of social media platforms at the moment. So, to reduce political polarization, for example, it might be beneficial to present people with both left- and right-wing ideas. This would increase the ties between these groups, which can lead to more understanding and leaves less room for extreme opinions (see again Feldman et al. for an interesting model on this topic).
In practice?
Although the conceptual explanation above seems reasonable, it turns out to be rather complex to simulate large-scale social networks, based on (the relatively simple) social phenomena such as confirmation bias. For instance, there is not even an agreed-upon model that describes how a social media network emerges in the first place. This makes it difficult to investigate whether echo chambers are a consequence purely of the organization of the platform and whether specific changes in the social network structure influence the creation of echo chambers.
Instead of an overarching model to describe complex collective behavior on all social media platforms, researchers have come up with many models that each describe the effects of echo chambers via some model for opinion dynamics. A research article where different opinion dynamics models are compared is Terizi et al., where a total of 26 models have been studied that describe how aggression spreads over a network.
We are currently working on developing a mathematical model which can be used it to assess whether a social media network contains or is an echo chamber, by comparing it with well-known (from literature) echo chambers. We are mainly interested in questions like: What are early warning signs of echo chamber formation? How should the network change to prevent the formation of echo chambers? What concrete measures can social media platforms implement to depolarize communities?
Applications on Reddit
Let us dive a bit deeper into one of these questions. While it is not clear how to specifically characterize an echo chamber, there are some properties of networks that are often used to determine the likelihood of echo chambers being present in a network. For example: the general opinion of a group of users, how often different groups interact with each other, and how much users within a group interact among themselves. If all these properties are above certain threshold values, we can say that there is a significant chance of an echo chamber being present.
While all these metrics can be derived from a network of users interacting with each other, it is difficult to quantify the general opinion of a group of users from social media data. Real-life data (for instance from Reddit) consists mainly of posts and comments, both with properties like an author, the amount of likes/dislikes, and a time stamp. The challenge is to construct a user network from these comment chains, to be able to analyze the network in terms of how likely it is that there are echo chambers at play.
An example of how this could work is described below, and shown in the picture on the right. On the right, you can see two parts of such a comment network, with at the top a post, directly beneath comments on that post, and below those comments to the first comments. The user network (on the left) is constructed as follows: each time one user comments on another user’s post or comment, we draw a directed edge (connection) from the first user to the other user.
For example, the orange and pink users both commented on the black user’s post, so we draw edges from the pink and orange user to the black one. We also note that the black user commented on the orange user’s post, so we draw that edge the other way around too. The remaining part of the user network is drawn analogously.
Although it may seem like a simple construction, in practice such a user network will contain an immense amount of nodes and edges, due to the number of posts that are being posted on social media. This means that analyzing such a user network is very challenging. But given this user network we can, for example, try to distinguish communities and compute the metrics we discussed at the start of this section.
Given lots and lots of comment networks, sampled from social media over a long time period, we can validate a model that dictates at what time a new post would be created and how many comments it would attract. If this model is sufficiently accurate in predicting the structure of these networks consisting of posts and comments, we could see what happens if we for instance diminish the influence certain very popular users have, or if we purposefully bring different communities closer together by presenting them with more content from outside their echo chambers. The effects that these changes would have, could be analyzed on the user network, which is constructed from the comment network that our model produces. In this way, we hope to shed some light on what the most important factors are in forming and keeping an echo chamber in place so that we know how to stop their emergence or at least halt their growth.
Outlook
To summarize, echo chambers are a real problem in the online world, and we can see them starting to change the offline world as well, through for example increased polarization and extremism. We believe that network science could help find a solution to lessen the real-life impact of echo chambers. By characterizing them and understanding how they are formed we can start finding approaches to halt the formation of online echo chambers. To make a step towards this goal, we aim to investigate the evolution of echo chambers on social media by comparing real-life data with predictions obtained from mathematical models. Knowing what influences their emergence and growth can give rise to further research on the disruption of echo chambers. Once we can take concrete action on how to break them apart, we hope to see a society with less polarization where people are open to debate and listen to opposing views.
The featured image was made by Gerd Altmann for Pixabay.