“Do you ever look at someone and wonder ‘What is going on inside their head?‘ ” (Inside Out trailer). Disney Pixar’s Inside Out playfully personifies emotions and depicts how our brain works to create thoughts and actions. Inside the brain’s “headquarters”, five quirky characters -“Joy”, “Sadness”, “Fear”, “Disgust”, and “Anger”- work the control panel, steering your decisions and actions.
Inside Riley's mind, Joy keeps things upbeat, Sadness brings the feels, Fear panics regularly, Disgust raises an eyebrow, and Anger… well, he presses the BIG RED button when things get heated.
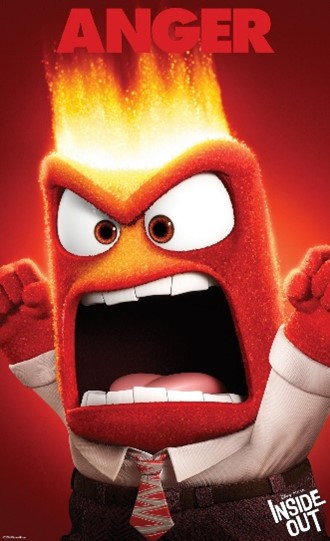
“Let’s see… this house stinks, our room stinks…”
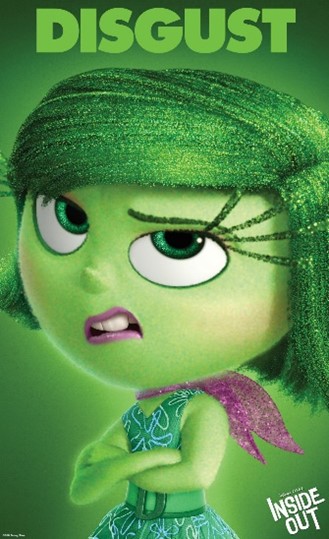
“Pizza is weird here”
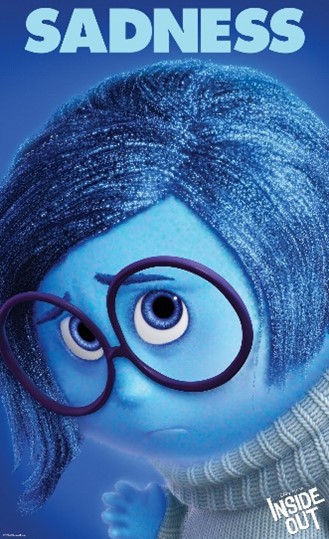
“Our friends are back home...”
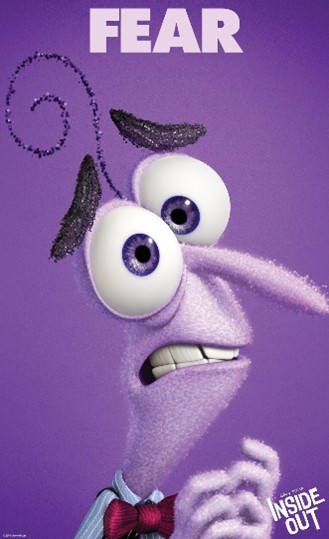
“All of our stuff is in the missing van!”
But in reality, the brain’s complexity goes far beyond what we might imagine. It operates non-stop, whether you’re sleeping or awake; making split second decisions, processing what's going on and adapting. It manages everything from keeping your heart beating to helping you solve a complex math problem.
The exact inner workings of the brain are still a mystery, but scientists from various disciplines are shedding light on it. Here, network science can play a valuable role! By studying the brain as a network, we can visualize its complexity and reveal how all the different components interact with each other.
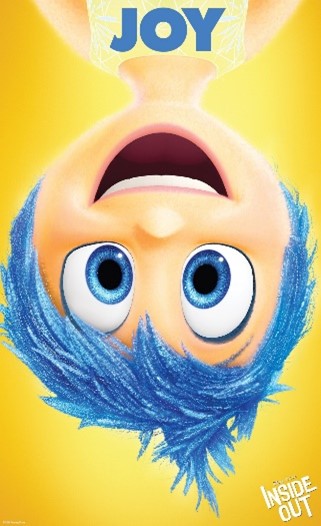
"Oh c'mon, it could be worse!"
One time in our lives where the development of the brain is especially fascinating is adolescence. We learn to navigate new social situations, to recognize cues from others and gradually develop an emotional awareness. Meanwhile our brains adapt and change consistently. Emotional awareness doesn't always come easy. Ever had one of those awkward moments where you drop a joke thinking it was comedy gold… only to be met with a stone-cold poker face? Yeah, emotional cues are tricky! For some it comes naturally. For others, it's more of a puzzle. But why is that? Why are some better at reading emotions than others?
Perhaps networks can be used to answer these questions. But before we explore how this can possibly be done, we need to take a step back and try to understand the brain through networks.
The brain as a network
The brain is a huge and complicated maze and "you can get lost in there!", as Sadness makes us aware. However, the design of this inner world is elegant and well-structured. In Inside Out's brain control room, this inner world consists of islands; an "Honesty Island", "Family Island", and even a "Goofball Island". All these components shape your personality. Beyond that is the abyss of the subconscious and even a theme park representing imagination!
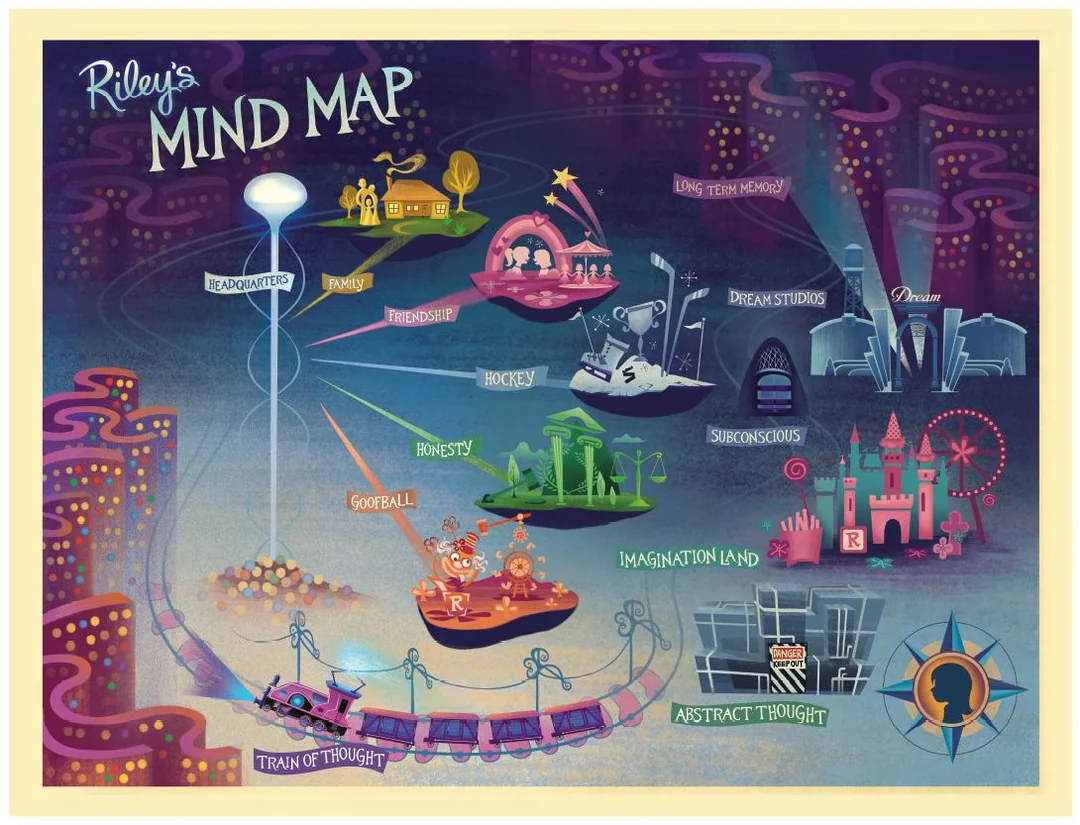
A fan-made mind map of Riley's mind in Disney Pixar's Inside Out posted on Reddit
What happens when you need to make a decision? Say you stole a candy from your mother's hidden stash. Well, your Goofball Island and Honesty Island have to work together to choose what to do next; do you honestly tell mom what happened, do you sneakily enjoy the candy with friends for some extra fun, or maybe both? You might visit Imagination Island to think of a creative solution. And once you decide, it has to be communicated back to headquarters. A lot more goes into a decision like this than you may anticipate!
How do all these components in the brain communicate with each other? How can Goofball Island, Honesty Island and Imagination Island work together and report back to headquarters? Well, the answer is the "Train of Thought", racing through your mind and delivering supplies like daydreams, facts, opinions, and of course, memories. This train allows all the parts of the brain to work together. If we look carefully, we can recognize the structures on islands as nodes and the tracks that the Train of Thought uses as edges. There it is, we've just constructed a network of the brain.
This is similar to how neuroscientists translate a brain to a network. A common way to look ‘inside’ someone’s body is an MRI scan, showing the structure of the brain. But structure alone doesn't tell us how the brain works. For that, we need something more: the functional MRI (fMRI).
In Inside Out terms, an fMRI measures the transport of goods between train stops. In a neurological sense, the fMRI shows how oxygen is transported between brain regions. When a brain region becomes active—say, to process a thought or emotion—it needs energy, which comes in the form of oxygen carried by red blood cells. The fMRI picks up on this by detecting changes in the magnetic field around the blood vessels, indicating where oxygen is being transported. By capturing many images of the brain over time we can create a dynamic picture of activity in different regions of the brain. [1]
Now here’s where the network comes in: if two brain regions show activity at the same time, we assume they’re functionally connected. We represent these regions as nodes, and their synchronized activity as edges [9]. We've constructed a functional network of the brain, a map that visualizes how different regions interact.
How is a brain network structured?
What can this map reveal about how the brain operates? Are all brain networks wired the same way, or are some better organized than others? Let us look at "efficiency" of the brain; ideally, there is a connection between every region in the brain to ensure short and quick communication. However, each connection consumes energy and there is limited energy available. So the network's structure is essential to how efficiently and effectively it operates. To assess this efficiency, we can use network measures. In particular, there are two characteristic features of brain networks that stand out: modularity and centrality.
Let’s begin with modularity. This measure refers to the partitioning of the network into smaller building blocks, also known as modules. As you may know, the brain is split into various areas; while one may be responsible for language, another may drive your memory, or motor control. Each building block specializes in a different cognitive task and blocks effectively work together for you to function. In our network, such a building block consists of several nodes. But do these nodes mostly communicate within their group or do they have many connections with other modules? In other words, to what extent do nodes communicate within their own 'community'?
To quantify this, there are two sub-components of modularity: segregation and integration. Segregation measures the level of internal connectivity within the brain’s modules. Integration quantifies how much these modules are connected to each other. [4]
Through the lens of Riley's mind, the brain's modules are islands. The islands are easily traversable, corresponding to a high segregation. In other words, it’s easy to travel on one island as there are many paths and roads. However, the islands are only weakly connected via only one track through which traffic is limited, corresponding to a low integration. Therefore, in this analogy of Inside Out, the islands are well-connected brain modules that barely communicate with each other, that is, work mostly independently.
During the early ages of adolescence, the brain is observed to become more modular. This means that modules become more specialized, corresponding to an increased segregation. As a result, such modules can process information between each other more efficiently with less connections, denoting a decreased integration. Scientists explain this change in the network as a shift from an inefficient and energy consuming system, to one that is efficient and capable of handling the complex tasks of adulthood; an important milestone in cognitive and emotional development. [8]
To illustrate this development, consider Riley’s brain. If we were to look at her brain over the years, we would see that as she matures, and more memories form, the islands become increasingly specialized, with more efficient and better transport systems, increasing segregation. Simultaneously, the infrastructure of the railway tracks between the islands is improved by defining a single better maintained track between each island, making global transport more efficient by using less tracks. These changes would occur gradually over her teenage years, transforming Riley's brain to a more efficient one day-by-day.
The second characteristic feature of brain networks is centrality. This measure indicates the importance or influence of a node within a network. Central nodes are critical to coordinating communication within and across the brain modules [5]. In terms of Inside out, think of a central node as the central station of Imagination Island. As every thought requires some degree of imagination, every processed thought has to go through Imagination Island, and more specifically through its central station. Hence the station is crucial for efficiency and it is thus a central node.
Measuring the brain mathematically
We would like to measure integration, segregation and centrality of the brain. To do this, we can extract information from the brain network we have constructed.
One way to measure integration in a network is using the measure "global efficiency". Integration determines how well modules are connected to each other, so a good measure would be based on how easily you can get from each node to every other node. If this is easy for many pairs of nodes, the modules are globally well interconnected , meaning we have high "global efficiency".
One way of measuring segregation is by the measure "local efficiency". The local efficiency determines the efficiency of communication between the immediate neighbors of a node in case that node is removed.
Lastly, we have the centrality which we measure through ‘leverage centrality’. There are many ways to measure centrality. Leverage centrality, however, is a specific centrality measure for brain networks. It considers the connectivity of a node relative to the connectivity of its neighbors.
An example network
Even if you did not look at the technical details, let us look at an example network:
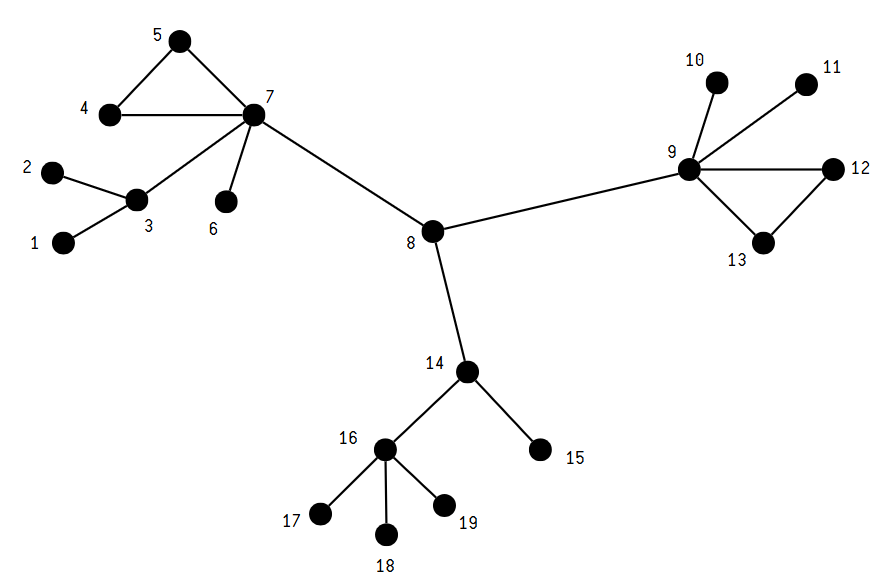
An example network
For global efficiency (integration), observe that node 7 exhibits a high efficiency (approximately ), since it communicates with many nodes of the network in just a few steps. In contrast, we can consider node 19, which is located at the edge of the network with a single connection (to node 16). This results in it not being able to communicate with other parts of the network as easily and efficiently as node 7, and thus, a lower efficiency (approximately
). Node 7 contributes high integration, while node 19 drags the network's integration down.
For local efficiency (segregation), look at node 4. All of node 4's neighbors are interconnected among themselves, meaning that it is possible to find paths between them without passing through node 4. In other words, within this part, the network can easily compensate if node 4 would go missing. It exhibits a high local efficiency (of value ), indicating high segregation. In contrast, the neighbors of node 16 are not connected to each other at all. So, if node 16 was to be removed, there would be no alternative paths for communication between them. As a result, node 16 exhibits a low local efficiency (of value
), indicating low segregation.
Lastly, we look at leverage centrality. Node 16 interacts with other nodes, each with few neighbors. Thus, in its local area, node 16 exhibits a higher influence; or, in other words, a higher leverage centrality (being approximately ). To put it into perspective, node 3 has a lower leverage centrality of
. It has a certain degree of influence compared to some of its neighbors such as node 1 and 2. However, it also has a well-connected neighbor, namely node 7, which results in the lower value. Finally node 6 which has a negative leverage centrality of
. This means node 6 is connected to neighbors that are better connected than itself. Hence, it has a lower influence and thus lower leverage centrality.
Having seen the example network and the measures, we can now quantify the development of a patient’s brain network.
What does this say about emotion recognition?
With this ability to quantify brain development, we can track how the brain matures over time. By analyzing the three network measures in patients of different ages, we can identify trends that reflect the brain’s developmental progression. Alongside this changing network during maturation, it is not unreasonable to think that the tasks and skills we are capable of performing and how well we are able to perform them change accordingly. Hence opening the opportunity to explore the connections between brain development and cognition throughout adolescence.
In fact, this has been the very same thought that has inspired many studies. Some studies have focused on how certain regions of the brain perform specific tasks and how the brain networks are involved in specific cognitive abilities such as motor skills, sensory aspects, attention, and memory systems. Other studies (some even based on network analysis) investigate the development of the performance of these abilities and skills [7].
An interesting area of research could be to investigate how the development of the whole brain corresponds to a complex yet very crucial task: emotion recognition. Hence, taking a more hollistic approach. Most notably, the study could focus on measuring the simultaneous development of both the brain and emotion recognition capabilities. This approach addresses a current gap in research by directly linking neural maturation with the ability to recognize emotions during adolescence.
Emotion recognition plays a crucial part in our lives. Adolescence is a time when who we have social contact with drastically changes. During adolescence, individuals shift from parental dependence to diverse social connections. This transition exposes them to increasingly complex emotional experiences, requiring adaptation and refined emotional interpretation [2]. Our ability to recognize emotions and accurately decode facial cues enhances social interactions, positively impacting relationships, academic performance, and future career success [10].
Emotion recognition plays a critical role in how we navigate social interactions, much like how Riley's parents try to determine her current mood. Is Anger taking the rails in her headquarters, eyebrows shaping into a frown, voice getting louder, or is Sadness making the eyes get watery? Not only does it become important to identify which character takes the lead in another person's headquarters but also to respond quick enough. One wrong move or one delayed action creates many more problems. Thus, the ability to quickly and accurately recognize emotions becomes crucial.
What's next?
The brain is the body's most complex organ, and its intricacy has enormous implications on what life means. Over the years, network science has helped fields like neuroscience and neurology in understanding the functioning of the brain and paved the way to revolutionary breakthroughs in these fields by providing an alternative perspective. This has assisted in solutions ranging from diagnosis or treatment of certain disorders, and more effective surgeries, to individually tailored education, and much more [6]. However, there are still many topics to be explored through a network lens.
Shedding light on the development of the brain network in relation to emotion recognition can bridge the gap between neuroscience and emotional development. This offers a fresh perspective on adolescent maturation and may help to identify deviations from the expected neurotypical trajectory of cognitive and emotional growth. By integrating network analysis with developmental neuroscience, new insights that contribute to both theoretical understanding and practical applications in emotional development could be uncovered.
Imagine, for example, being able to detect when someone's railway tracks between islands are broken, blocking the train of thought from efficient travel; perhaps explaining delays in task performance. Or imagine recognizing when one of the emotion characters is missing from the brain's headquarters, explaining why this person cannot recognize that particular feeling in others. These are just a few of the scenarios we cannot yet explain. Ultimately, studies exploring this topic through the lens of networks could help understand how the emotional "crew" works together in someone's brain- or at least how Riley can learn to spot when Anger’s about to slam the BIG RED button in someone else’s head… and maybe hold back that joke when all she’s getting is a stone-cold poker face.
References
[1] | Gary H Glover. Overview of functional magnetic resonance imaging. Neurosurg Clin N Am, April 2012. |
[2] | Maria Guarnera, Zaira Hichy, Maria I. Cascio, and Silvia Carrubba. Facial expressions and ability to recognize emotions from eyes or mouth in children. Europe’s Journal of Psychology, 11(2):183–196, 2015. |
[3] | Jonathan H. Burdette Satoru Hayasaka Karen E. Joyce, Paul J. Laurienti. A new measure of centrality for brain networks. PLoS ONE, August 2010. |
[4] | Mikail Rubinov and Olaf Sporns. Complex network measures of brain connectivity: Uses and interpretations. NeuroImage, 52:1059–1069, 9 2010. |
[5] | Olaf Sporns. Graph theory methods: Applications in brain networks. Dialogues in Clinical Neuroscience, 20:111–120, 2018. |
[6] | Cornelis J. Stam. Modern network science of neurological disorders. Nature Reviews Neuroscience, 15:683–695, 2014. |
[7] | Kaustubh Supekar, Mark Musen, and Vinod Menon. Development of large-scale functional brain networks in children. PLoS Biology, 7(7):e1000157, 2009. |
[8] | Ursula A. Tooley, Anne T. Park, Julia A. Leonard, Austin L. Boroshok, Cassidy L. McDermott, Matthew D. Tisdall, Dani S. Bassett, and Allyson P. Mackey. The age of reason: Functional brain network development during childhood. Journal of Neuroscience, 42:8237–8251, 11 2022. |
[9] | Haixian Wang Xuan Li. Identification of functional networks in resting state fmri data using adaptive sparse representation and affinity propagation clustering. Frontiers in Neuroscience, October 2015. |
[10] | Barbra Zupan and Michelle Eskritt. Facial and vocal emotion recognition in adolescence: A systematic review. Adolescent Research Review, 9:253–277, 2024. |
Cover photo and character images by Disney/Pixar. Found on this page and this page, respectively.